Data Science Visionary Convinces on Transformative Power of Artificial Intelligence—But How Much Can We Trust it?
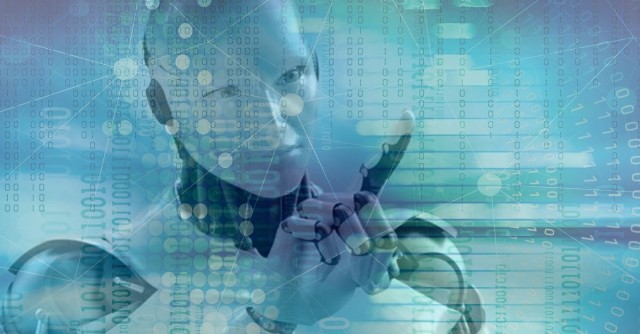
“I think artificial intelligence is going to change everything 180 degrees.” Mark Cuban, billionaire investor, owner of the NBA’s Dallas Mavericks and a regular on the TV show “Shark Tank,” made this statement in a recent interview with Real Vision Television. He then added that changes seen by artificial intelligence would “dwarf” the advances that have been seen in technology over the last 30 years or more, even the internet.
We all know that artificial intelligence (AI) is among the dominant topics in the financial markets today, seemingly promising to provide numerous benefits to any capital markets institution that embraces it. The potential advantages include improved operational and cost efficiencies, enhanced client services, improved data and analytics, increased profit and revenue generation, and—the holy grail of every fund manager—better returns.
Validating the tremendous potential for AI application in the capital markets is the fact that, by the end of 2017, it is estimated that financial firms potentially spent more than $1.5 billion on AI-related technologies and, by 2021, potentially $2.8 billion, representing an increase of 75% over a four-year period.
At a basic level, artificial intelligence (AI) is about machines that are designed to complete tasks that would normally require human intervention. These machines “learn” as they encounter new tasks or situations. They access data, apply algorithms to this data, and then train themselves to deduce valuable insights and make decisions based on the underlying datasets.
I agree that artificial intelligence—and machine learning, the process upon which AI is built—could transform and empower finance by significant measures, but AI is still very much a maturing industry and the following question stands out: Can machines be trusted?
At our NEXT conference held in October 2017, data science visionary Dr. Vasant Dhar – a professor at NYU’s Stern School of Business and the Center for Data Science, founder of SCT Capital Management, and editor-in-chief of Big Data Journal – presented his insights and analysis on this very question. He also provided profound perspectives on the growing AI phenomenon, the challenges it still faces, and what it means in the financial services domain. I’d like to share some of his thinking, which I found illuminating on the case of AI in the investment landscape.
The AI Conundrum
Dr. Dhar upholds that the issue of when we should trust AI machines with making decisions and when we should not is predicated on the conundrum that artificial intelligence, and thus machine learning, can, in theory, add tremendous value to society (think of accelerated treatment of diseases) but that there are also many ways it can fail—and in some cases the consequence of an error could be significant (think of a driverless car failing to detect pedestrians in Times Square).
Dr. Dhar’s large body of work includes creating a framework, which he calls the Automation Frontier, to help gauge which decisions we could currently feel comfortable with a machine making and which should be kept with a human—think of it as a model of trust. The framework differentiates tasks along two independent dimensions: predictability and cost per error. The former, as Dr. Dhar explained, is based on a statistical technique called predictive analytics, which encompasses a variety of data, statistical algorithms and machine learning techniques to identify the likelihood of future outcomes. In the framework, the order of predictability ranges from “random” (low predictability) to “sure thing” (high predictability). For example, if you ask me who is going to win the Super Bowl in February, well, I don’t know, so any answer I give would be completely random. But if you ask me if I am going to have lunch tomorrow, well yes, that’s more of a sure thing. He applies his predictive analytics in a number of areas, including financial markets, healthcare, and education.
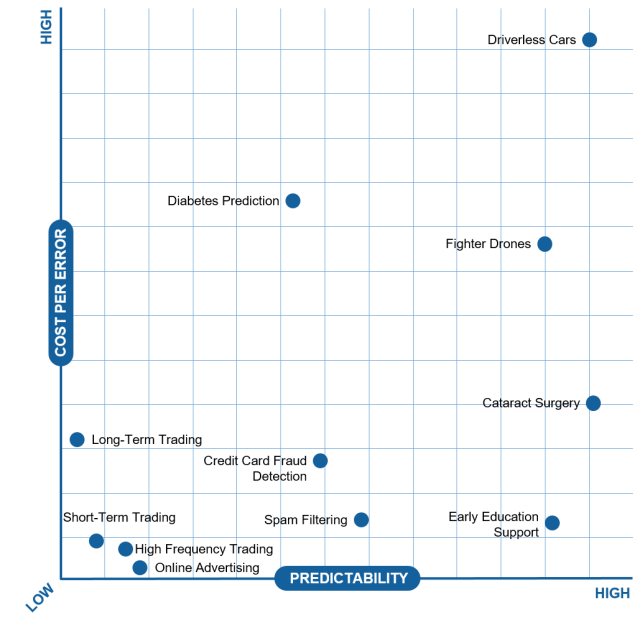
The other representation on Dr. Dhar’s framework, cost per error, is about the consequences of a mistake. For example, a combat drone falls high on the predictability scale because drones have achieved strong reliability over the time of their evolution and have even become commonplace in individual life. But what if a combat drone is sent on a mission and mistakes a hospital for an arms depot? So, this is an example of an item that is plotted on the higher predictability, higher cost of error spectrum of the framework. The figure above shows the automation frontier, where how problems can move to the right on the map through more data and better algorithms – which enhance predictability, and move down or up due to decreasing or increasing costs of error due to factors such as regulation.
Where does asset management fall on the map at the moment? For now, Dr. Dhar plots the investing landscape in the “low predictability, low cost per mistake” end of the framework, breaking it out into three investment strategies: high-frequency trading, short-term trading and long-term trading. For purposes of this discussion, I am going to set aside high-frequency trading because asset management is more about looking for the best trades in the longer term—whether it be for a period of months or years—and not about making money from speed of action.
Dr. Dhar ascribes low predictive power to AI engines in the investment landscape because the problem is inherently “noisy”—there are too many exogenous “shocks” to the system in terms of inherently unpredictable events such as central bank actions, terrorism, surprise earnings, scandals, and more. These AI engines, after analyzing everything from market prices and volumes, macroeconomic data and any exogenous shocks or events in the financial markets, make their own market predictions and consider the best course of action based on what they self-learned. But their limited degree of “explainability” in terms of providing simple rationales for their decisions can impede trust and inhibit money managers from trusting AI completely—in the sense of letting a machine make 100% of the decisions without any human supervision or intervention. It is also challenging to elucidate and enumerate for a client how and why the AI investment model would perform under different market conditions. While hedge funds that use AI models to help make trade choices—known as systematic hedge funds—have performed well over the last two years, it is important to observe their behaviors over longer periods. Dr. Dhar has written elsewhere that it might be easier to find a good robot relative to a good human investor if sufficient performance data are available.
Dr. Dhar also commented that the technology for AI in asset management is maturing significantly, particularly as the collaboration between financial services companies and fintech increases. He also noted that numerous fintech startups are joining the scene with very niche AI technology solutions.
Additionally, Dr. Dhar stressed that it is important to recognize that the AI engine can statistically evaluate large quantities of data, interpret signals, and observe market structures much more robustly than any human can. And as the algorithms advance more and more, the AI machine will do a much better job of automatically self-correcting and learning from its mistakes.
So as to the question of whether we can trust machines to make investment decisions as good as or better than humans, Dr. Dhar says the answer is, “we are getting closer to yes”.